If you are interested to learn about the Python nth fibonacci Number
OpenCV is the huge and open-source library for image processing, machine learning and computer vision. It is also playing an important role in real-time operation. With the help of the OpenCV library, we can easily process the images as well as videos to identify the objects, faces or even handwriting of a human present in the file. We will only focus to object detection from images using OpenCV in this tutorial. We will learn about how we can use OpenCV to do object detection from a given image using a Python program.
Object Detection
Basically, object detection is a modern computer technology that is related to image processing, deep learning and computer vision to detect the objects present in an image file. All the technologies used in the Object detection technique (as we mentioned earlier) deals with detecting instances of the object in the image or video.
Object Detection using OpenCV
We have learned about object detection in the previous section, and in this section, we will learn that how we can do object detection in an image or video using the OpenCV library. We will first import the OpenCV library in the Python program, and then we will use functions to perform object detection on an image file given to us. But, before using and importing the library functions, let’s first install the requirements for using the Object detection technique.
In this tutorial, we will use the Haar cascade technique to do object detection. Let’s learn in brief about the Haar cascade technique first.
Is OpenCV used for object detection?
OpenCV has a bunch of pre-trained classifiers that can be used to identify objects such as trees, number plates, faces, eyes, etc. We can use any of these classifiers to detect the object as per our need
How do you identify an object in Python?
In Python, the built-in functions type() and isinstance() help you determine the type of an object.
- type(object) – Returns a string representation of the object’s type.
- isinstance(object, class) – Returns a Boolean True if the object is an instance of the class, and False otherwise.
How do you make an object detection in Python?
Steps to download the requirements below:
- Run The following command in the terminal to install opencv. pip install opencv-python.
- Run the following command to in the terminal install the matplotlib. pip install matplotlib.
- To download the haar cascade file and image used in the below code as a zip file click here.
What is the use of OpenCV in Python?
OpenCV is a great tool for image processing and performing computer vision tasks. It is an open-source library that can be used to perform tasks like face detection, objection tracking, landmark detection, and much more. It supports multiple languages including python, java C++
Haar cascade:
Basically, the Haar cascade technique is an approach based on machine learning where we use a lot of positive and negative images to train the classifier to classify between the images. Haar cascade classifiers are considered as the effective way to do object detection with the OpenCV library. Now, let’s understand the concept of positive and negative images that we have discussed earlier:
- Positive images: These are the images that contain the objects which we want to be identified from the classifier.
- Negative Images: These are the images that do not contain any object that we want to be detected by the classifier, and these can be images of everything else.
Requirements for object detection with Python OpenCV:
We have to install first some important libraries in our system as it is an important requirement for doing object detection tasks. We have to install the following libraries into our system as the requirement for performing object detection:
1. Installation of OpenCV library:
First and foremost, the requirement to perform object detection using the OpenCV library is that the OpenCV library should be present in our device so that we can import it into a Python program and use its object detection functions. If this library is not present in our system, we can use the following command in our command prompt terminal to install it:
pip install opencv-python
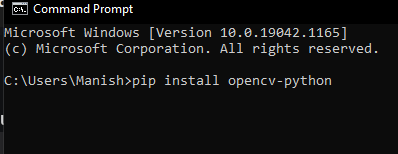
When we press the enter key after writing this command in the terminal, the pip installer in the command prompt will start installing the OpenCV library into our system.
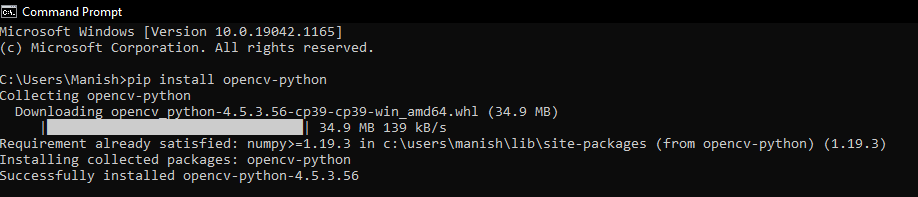
As we can see that, the OpenCV library is successfully installed in our system, and now we can import it into a Python program to use its functions.
2. Installation of matplotlib library:
Matplotlib is very helpful in the opening, closing, reading etc., images in a Python program, and that’s why the installation of this library for object detection becomes an important requirement. If the matplotlib library is not present in our system, we have to use the following command in our command prompt terminal to install it:
pip install matplotlib

When we press the enter key after writing this command in the terminal, the pip installer in the command prompt will start installing it into our system.

As we can see that, the matplotlib library is successfully installed in our system, and now we can import it into a Python program to use its functions for opening, reading etc., images. We have installed all the required libraries for performing object detection, and now we can move ahead with the implementation part of this task.
Implementation of Object detection in Python:
In this part, we will write the Python programs to do the object detection and understand the implementation of it. We will use the following image in our Python program to perform the object detection on it:
Opening the Image
We will first open the image given above and create the environment of the picture to show it in the output. Let’s first look at an example program to understand the implementation, and then we will look at the explanation part.
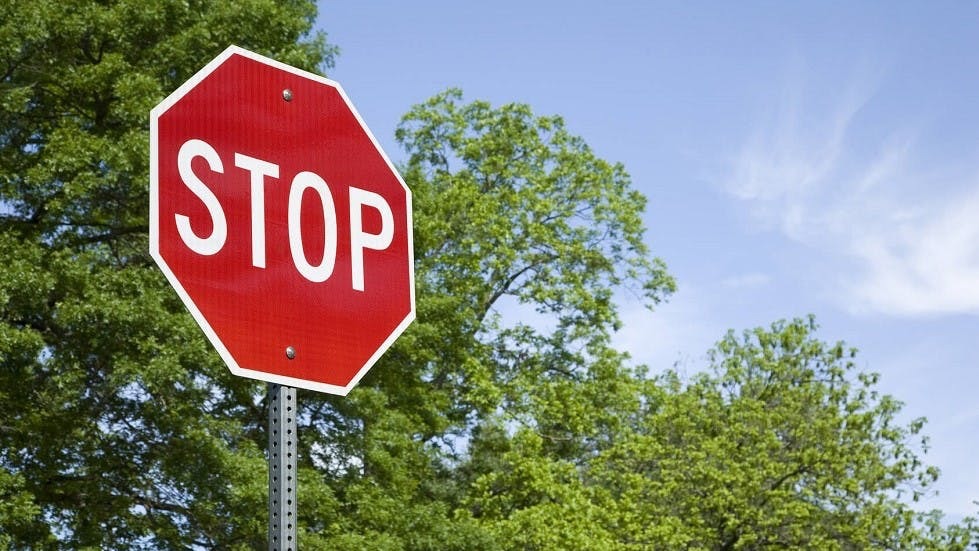
Example 1: Opening the image using OpenCV and matplotlib library in a Python program:
# Import OpenCV module import cv2 # Import pyplot from matplotlib as pltd from matplotlib import pyplot as pltd # Opening the image from files imaging = cv2.imread("opencv-od.png") # Altering properties of image with cv2 img_gray = cv2.cvtColor(imaging, cv2.COLOR_BGR2GRAY) imaging_rgb = cv2.cvtColor(imaging, cv2.COLOR_BGR2RGB) # Plotting image with subplot() from plt pltd.subplot(1, 1, 1) # Displaying image in the output pltd.imshow(imaging_rgb) pltd.show()
Output:
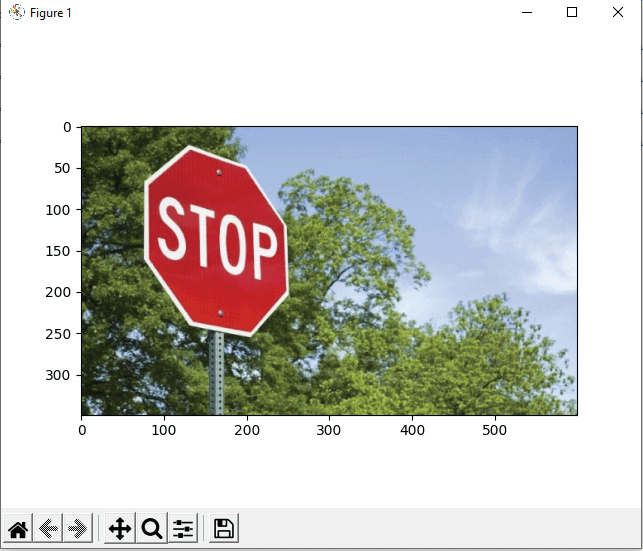
Explanation:
First, we have imported the OpenCV (as cv2) and matplotlib (as plt) libraries into the program to use their functions in the code. After that, we have opened the image file using the imread() function of cv2. Then, we have defined the properties for the image we opened in the program using the cv2 functions. Then, we subplot the image using the subplot() function of plt and giving parameters in it. In last, we have used the imshow() and show() function of the plt module to show the image in the output. As we can see in the output, the image is displayed as a result of the program, and its borders have been sub-plotted.
Recognition or object detection in the image
Now, we will use the detectMultiScale() in the program to detect the object present in the image. Following is the syntax for using detectMultiScale() function in the code:
found = xml_data.detectMultiScale(img_gray, minSize = (30, 30))
We will use a condition statement with this function in the program to check if any object from the image is detected or not and highlight the detected part. Let’s understand the implementation of object detection in the image through an example program.
Example 2: Object detection in the image using the detectMultiScale() in the following Python program:
# Import OpenCV module import cv2 # Import pyplot from matplotlib as plt from matplotlib import pyplot as pltd # Opening the image from files imaging = cv2.imread("opencv-od.png") # Altering properties of image with cv2 imaging_gray = cv2.cvtColor(imaging, cv2.COLOR_BGR2GRAY) imaging_rgb = cv2.cvtColor(imaging, cv2.COLOR_BGR2RGB) # Importing Haar cascade classifier xml data xml_data = cv2.CascadeClassifier('XML-data.xml') # Detecting object in the image with Haar cascade classifier detecting = xml_data.detectMultiScale(imaging_gray, minSize = (30, 30)) # Amount of object detected amountDetecting = len(detecting) # Using if condition to highlight the object detected if amountDetecting != 0: for (a, b, width, height) in detecting: cv2.rectangle(imaging_rgb, (a, b), # Highlighting detected object with rectangle (a + height, b + width), (0, 275, 0), 9) # Plotting image with subplot() from plt pltd.subplot(1, 1, 1) # Displaying image in the output pltd.imshow(imaging_rgb) pltd.show()
Output:
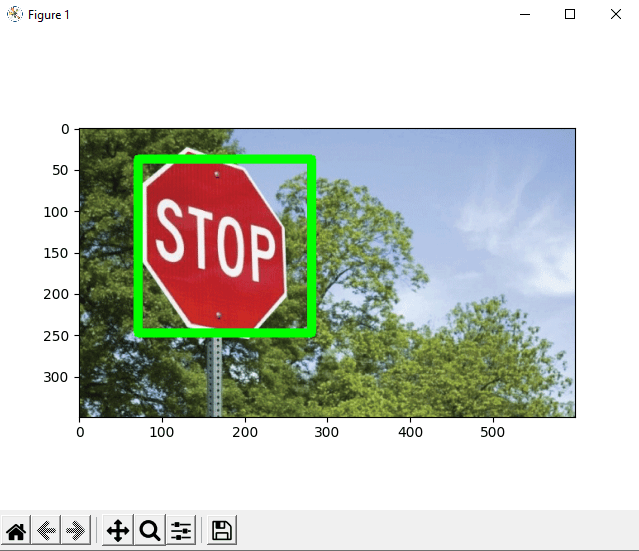
Explanation:
After opening the image in the program, we have imported the cascade classifier XML file into the program. Then, we used the detectMultiScale() function with the imported cascade file to detect the object present in the image or not.
We used if condition in the program to check that object is detected or not, and if the object is detected, we have highlighted the detected object part using for loop with cv2 functions. After highlighting the detected object part in the image, we have displayed the processed image using the plt show() and imshow() function.
Moving Object Detection using Frame Differencing and Summing Technique
As the name suggests, we can detect any moving object in a video using this technique. Basically, the main motive here is to do motion detection in videos.Moving object detection has a range of use cases ranging from surveillance to security. So, finding a technique that can be easily used in low computation devices is crucial. That is exactly what Thapa et al. have proposed in their paper. I recommend that you go through the paper as it is very readable.
They propose a method using which we can easily detect any moving object in a video. They employ the power of frame differencing and summing technique. Let’s know more about frame differencing and summing technique.
Frame Differencing and Summing Technique in Computer Vision
Frame Differencing and Summing Technique (DST for short) is a very simple yet effective computer vision technique. We can use it to know whether there are any moving objects in a video or not.
We know that a video consists of multiple consecutive frames. And these frames are made up of pixels which consist of colors (red, green, and blue). And these pixels are just values in the range of 0 to 255. 0 is completely black and 255 is white.
So, suppose that we take any two consecutive frames from a video. Now, let’s subtract the current frame from the previous frame. If they contain the same information (RGB color values), then the resulting frame will be completely black. But if the current frame consists of some newer information or pixel values, then we will see some sort of white patches after the subtraction. This tells us that something in the video has moved or changed position. This is a very simple concept. Yet we will use this as the basis for moving object detection in videos.
Let’s know about it in a bit more detail.
Steps for Moving Object Detection using Frame Differencing and Summing
Detecting moving objects using frame differencing and summing involves some simple steps. The authors have described it quite well in the paper. Let’s observe the block diagram which shows the complete procedure.
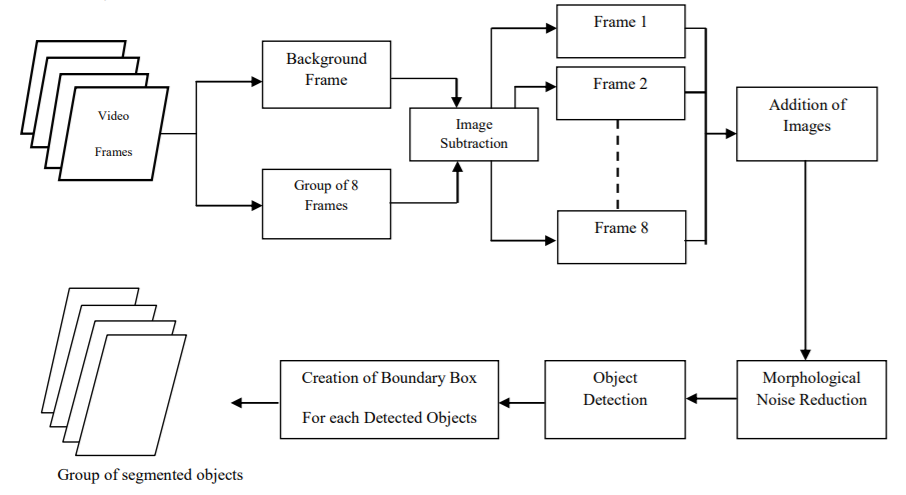
Figure 1 shows all the steps involved as a block diagram. These steps are very clearly explained in the paper as well.
If we can divide this whole process, then it can be described as the combination of two important processes which have their own steps in-turn. They are:
- Moving object detection and segmentation from the video frames.
- Drawing the bounding boxes around the detected moving objects.
In the following sub-sections, we will get to know about the two processes in more detail. We will also learn about the intermediate steps involved.
Moving Object Detection and Segmentation
The moving object detection and segmentation directly use the video frames that we are dealing with. This is also the process where we use frame DST (Differencing and Summing Technique). Moving further, let’s learn about the steps that we have to go through for moving object detection and segmentation from the video frames. These are a total of 7 steps. You will also find the steps in the paper. Still, let’s go through them here.
Step 1: Read 8 Consecutive Frames from the Video
First, we have to read 8 consecutive frames from the video. Now, if we take the case of Clip 1 that we saw before, then 8 consecutive frames will look something like this.
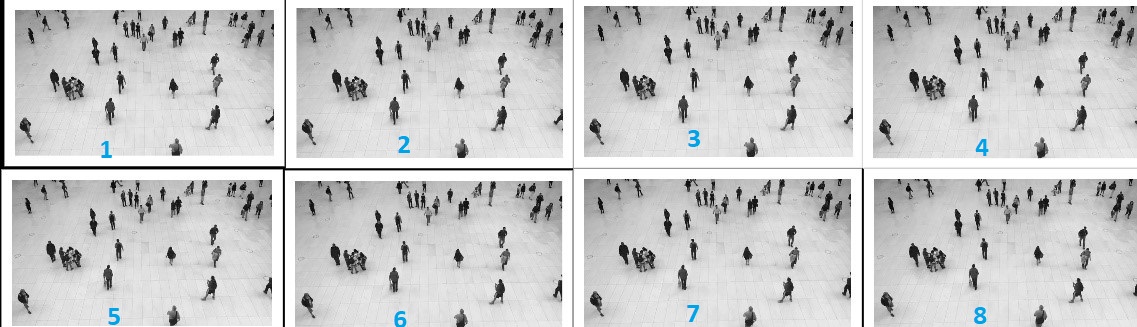
You can see all the 8 frames starting from top left corner. They all look almost the same.
Step 2: Convert the Frames into Grayscale
This is one of the important steps. We have to convert all the 8 frames into grayscale color format which will later help us with noise reduction and dilation. We will get into these details while writing the code.
Step 3: Frame Differencing
After every 8 consecutive frames, take the 8 frames and do frame differencing by subtracting the each of the frames from the background model. We will have get the background model before this. To get the background model, we will take a the median of certain number of frames. But how does the frame differencing look like? The following image will give you a good idea.
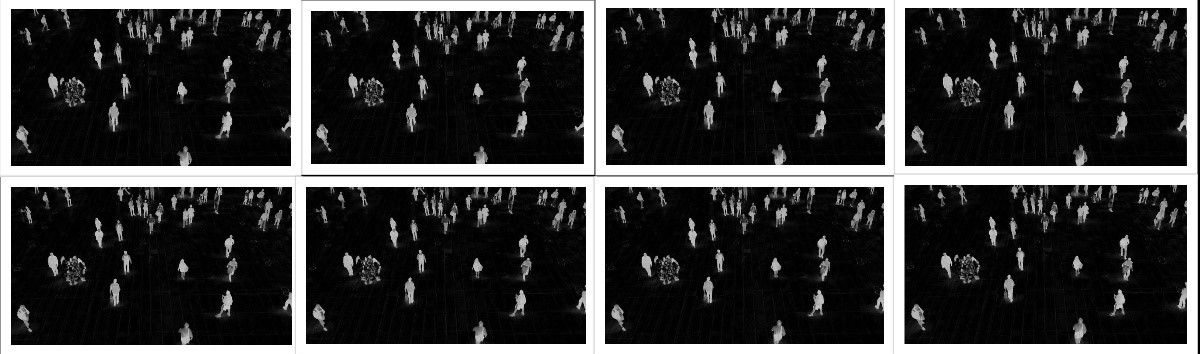
Figure 3 shows the frame differencing with the background model for each of the frames in figure 2. I hope that this makes things clear.
Step 4: Add the 8 Frames
After we get the frames by subtracting from the background model, we just add them.
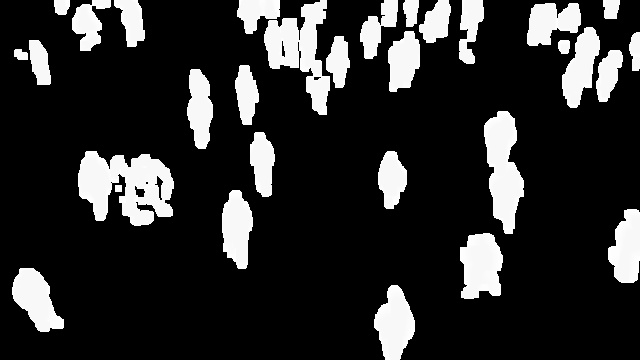
Figure 4 shows the result when we add all the 8 frames from figure 3.
Step 5: Fill the Gaps Inside the Objects
We need to fill the gaps so that the obtained objects look a bit more whole.
Step 6: Convert to Binary
Convert the image obtained in step 5 to a binary image by applying thresholding. Now we have a binary image out of the grayscale image.
Step 7: Remove Noise from the Resulting Image
We can apply a morphological operation to remove any extra noise. Morphological operations like dilations will help us with this. The above steps actually complete the detection and segmentation of the moving objects in a video. After that , we still have to draw the bounding boxes around the detected objects. That is the one of the important parts of the block diagram as well that we saw above.